Modelling the careers of cricket players
The Challenge:
To provide more accurate quantifications of current and future player batting abilities than traditional cricketing statistics, such as the batting average.
The Solution:
Using NeSI computing resources, researchers have developed and refined a model that uses a Gaussian process to measure and predict how the batting abilities of international cricketers fluctuate between innings.
The Outcome:
This new model can easily quantify differences in abilities and predict the real life impacts of selecting one player over another, all the while maintaining an intuitive cricketing interpretation, allowing for the results and implications to be easily digested by coaches and selectors.
Thanks to New Zealand’s recent run to the finals in the World Cup, cricket statistics have occupied the minds of many kiwis these last few weeks. Welcome to the world of University of Auckland researchers Oliver Stevenson and Brendon James Brewer.
“As a sport, cricket is a statistician’s dream,” says Oliver, a Doctoral Candidate at the University of Auckland’s Department of Statistics. “The game is steeped in numerous statistical and record-keeping traditions.”
Oliver’s statistical work mostly focuses on measuring player batting ability. Batting average is often used to measure this, where a higher average generally indicates better ability.
What a batting average doesn’t account for, however, is variation in ability, which can happen over the duration of a player’s career.
“Short-term changes in ability are observed during or within a single innings, due to factors such as adapting to the local pitch and weather conditions,” explains Oliver. “Long-term changes are observed between innings, over entire playing careers, due to the likes of age, experience and general changes in a player’s technique.”
To measure the long-term variations, the Auckland researchers have developed a model that uses a Gaussian process to measure and predict how the batting abilities of international cricketers fluctuate between innings.
A paper on this work, titled “Modelling Career Trajectories of Cricket Players Using Gaussian Processes”, was published as part of the conference proceedings from the 2018 Bayesian Young Statisticians Meeting (BAYSM 2018) at the University of Warwick in the UK, which Oliver attended last year.
The model requires a reasonable amount of computing power to fit, especially for players who have enjoyed long international careers. For players with long careers, it can take a long time (up to four days) to fit the model to their career data.
“NeSI allows us to run the model on hundreds of players simultaneously, rather than on one player at a time,” says Oliver. “Using NeSI allows us to analyse a larger number of players, in a shorter space of time.”
To build the model, they used Test career scores obtained from Statsguru, a cricket statistics database.
“As the model assumes that a player’s underlying ability is not influenced by the specific match scenario, it is best suited to longer form cricket, such as Test matches, where there is generally minimal external pressure on batsmen to score runs at a prescribed rate,” says Oliver.
When estimating player ability, the model takes into account a player’s recent form, as well as a number of match-specific factors, such as whether the match was played at a home or away venue.
For example, Figure 1 from their paper shows how the estimated batting ability of New Zealand’s current captain, Kane Williamson, has evolved over the course of his Test career.
Early in his career, Williamson's underlying ability is estimated to have been less than that implied by his career average of 53.38. However, an estimate of his current and future abilities suggest he is now a better batsman than his career average indicates.
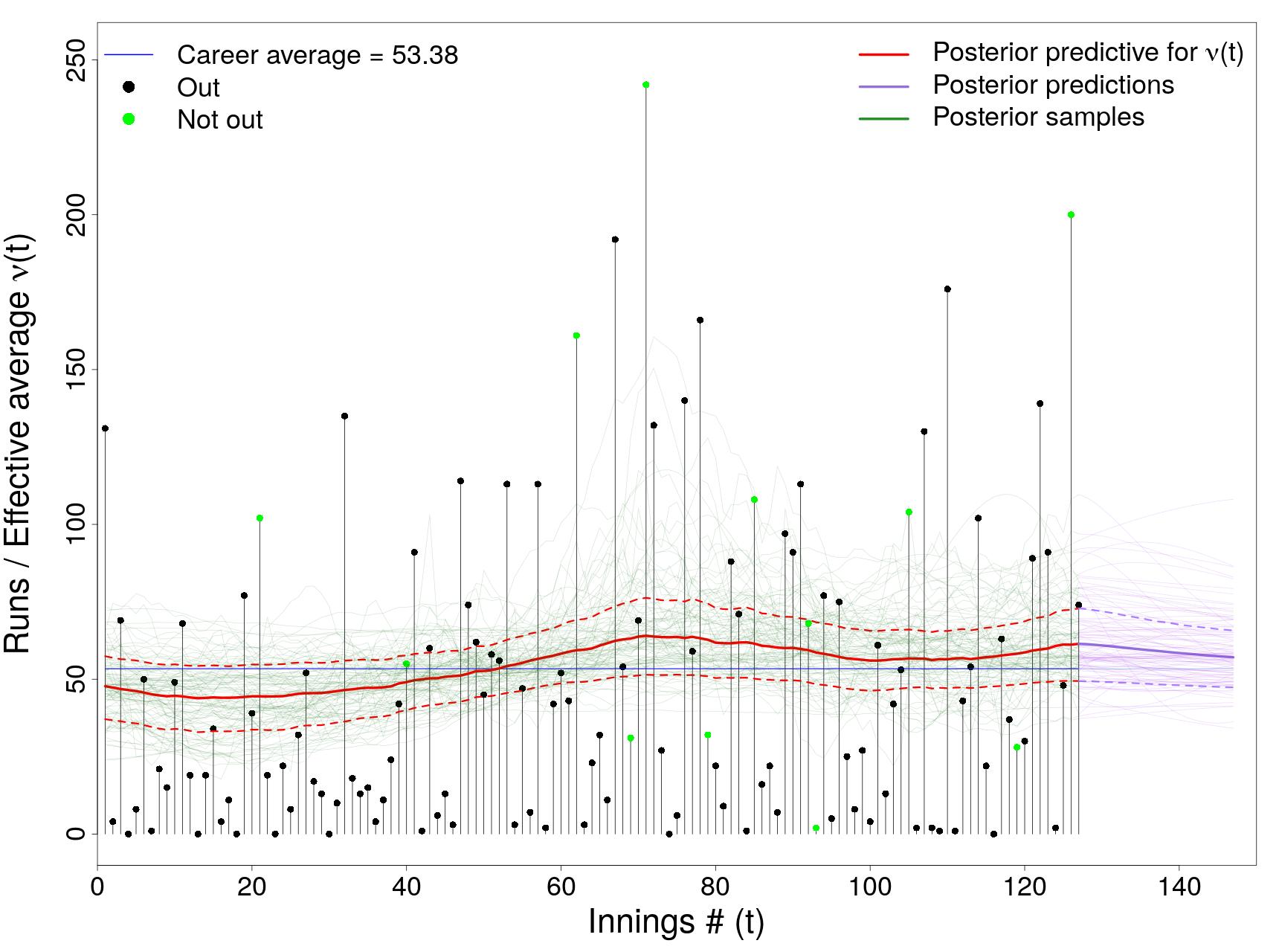
“This is not surprising, as it is a commonly held belief that many players need to play in a number of matches to ‘find their feet’ at the international level, before reaching their peak ability,” says Oliver. “Young players tend to begin their careers with some raw ability, which improves over time as a result of coaching, experience and other external circumstances. Eventually, players reach the peak of their career, after which ability tends to decline.”
Their model aims to provide more accurate quantifications of current and future player batting abilities than traditional cricketing statistics, such as the batting average.
“A major advantage of the model is that we are able to maintain an intuitive cricketing interpretation, allowing for the results and implications to be easily digested by coaches and selectors, who may have minimal statistical training,” says Oliver. “Additionally, we are able to make probabilistic statements and comparisons between players, allowing us to easily quantify differences in abilities and predict the real life impacts of selecting one player over another.”
Looking ahead, their next project will aim to fit a similar model to analyse the career trajectories of bowlers. NeSI will again be used to perform a large component of the computational work.
High performance computing (HPC) resources, like those provided by NeSI, have become an important tool for these researchers’ work, allowing them to tackle more complex challenges and collect more accurate results in a shorter period of time.
“While we could have “technically” fitted these models 10 years ago, it would have taken weeks or possibly even months to analyse the same set of players we have analysed in this paper,” says Oliver. “As HPC grows, so can the complexity of our models, which hopefully can translate to us being able to analyse and explain deeper aspects of the game.”
As for the World Cup game that held the focus of the cricket world earlier this month, Oliver was among the millions of fans who stayed up to watch the historic final. While the result wasn’t the outcome he’d been hoping for, he says the match was a “roller coaster of emotions” and one of the greatest he’s witnessed.
By the time the next World Cup rolls around, Oliver and his colleagues, powered by NeSI HPC resources, will have even more tools for analysing cricket and its athletes.
Publication:
Stevenson, O. G., & Brewer, B. J. (in press). Modelling career trajectories of cricket players using Gaussian processes. In Bayesian Statistics: New Challenges and New Generations – BAYSM 2018. Springer
- - - - - -
Do you have an example of how NeSI platforms or expertise have supported your work? We’re always looking for projects to feature as a case study. Get in touch by emailing support@nesi.org.nz.